Introduction
With the total contribution of AI to the global economy projected to reach $15.7 trillion by 2030, according to PwC Global, and generative AI demonstrating a remarkable 66% improvement in users' performance across three case studies, according to research at the Nielsen Norman Group, it's evident that artificial intelligence is reshaping industries and driving unprecedented growth. But what exactly distinguishes traditional AI from generative AI? Let's explore the definitions, applications, and key differences between these two subsets of artificial intelligence to understand their transformative impact across various domains.
Exploring Traditional AI: Limitations and Applications
Traditional AI, sometimes known as Weak AI or Narrow AI, is a subset of artificial intelligence that focuses on executing preset tasks using predetermined algorithms and rules. These artificial intelligence applications are designed to excel in a single activity or a restricted set of tasks, such as playing chess, diagnosing diseases, or translating languages.
Traditional AI is clever, but it may not necessarily be responsive. "Other examples of traditional AIs are voice assistants like Siri or Alexa, recommendation engines on Netflix or Amazon, or Google's search algorithm. These AIs have been trained to follow specific rules, do a particular job, and do it well, but they don’t create anything new," wrote Forbes.
There are plenty of useful applications for traditional AI. Some artificial intelligence spam filters, for instance, use predefined rules to isolate spam emails from your main inbox. However, traditional AI is only as effective as the data used to train the algorithm. It has limited efficacy in streamlining and optimizing your business.
Practical Applications of Traditional AI: Scenarios and Use Cases
Traditional AI systems often undergo training using large datasets that are annotated or labelled with specific attributes. During this training process, the system learns to recognize patterns within the data, enabling it to make predictions or generate outputs based on these learned patterns.
These systems use pattern recognition techniques to suggest movies, products, or music aligned with individual user preferences:
- Spam Filters: Email providers train their spam filtering algorithms on labelled datasets of spam and non-spam emails. These algorithms learn patterns in email content and sender behaviour to accurately classify incoming messages as either spam or legitimate.
- Autonomous Vehicles: Autonomous vehicles rely on extensive datasets of road conditions, traffic patterns, and sensor data to navigate safely. Traditional AI techniques, such as decision trees, are used to interpret sensor data and make driving decisions based on learned patterns.
- Fraud Detection: Financial institutions train their fraud detection systems on labelled datasets of past fraudulent transactions. These systems use pattern recognition algorithms to detect suspicious activities and flag them for further investigation.
- Language Translation: Language translation services like Google Translate use large corpora of translated text to train their NLP models. These models learn patterns in language structure and syntax to accurately translate text between different languages.
Introduction to Generative AI
Introducing Generative AI, a groundbreaking advancement in artificial intelligence that utilises neural networks, a type of machine learning algorithm, to create original content across diverse mediums. Neural networks are sophisticated algorithms inspired by the structure and function of the human brain. They analyse vast datasets to identify patterns and relationships, enabling them to generate various types of data, such as text, images, audio, videos, and 3D models.
- Generative AI builds upon the foundation of large language models (LLMs), which are trained on extensive amounts of text data using machine learning techniques. Leveraging this training, Generative AI algorithms predict subsequent words in sentences or paragraphs, facilitating the generation of new content.
- Notable examples of Generative AI applications include ChatGPT, DALL-E, and BARD, demonstrating their ability to produce a wide range of media forms, including text-based conversations and visual artworks.
- The popularity of Generative AI is increasing due to its capability to produce high-quality content anchored in its training data. From generating lifelike text and imagery to crafting complex code and simulations, Generative AI is a versatile tool with broad applications across industries and creative fields
Exploring the Transformative Power of Generative AI Across Industries
Generative AI, a transformative technology within artificial intelligence, finds diverse applications across numerous domains. Here's how it's reshaping various industries:
- E-commerce: Generative AI can be used to create virtual try-on experience for clothing and accessories, allowing customers to visualise how items will look before making a purchase. It can also generate product recommendations based on user preferences and browsing history, enhancing the shopping experience.
- Finance: In the finance sector, generative AI can assist with risk assessment and fraud detection by analysing transaction patterns and identifying anomalies. It can also generate financial reports, forecasts, and investment strategies based on market data and trends.
- Education: Generative AI can create personalised learning experiences by generating custom learning materials tailored to individual students' needs and learning styles. It can also simulate real-world scenarios for interactive learning and training purposes.
- Marketing and Advertising: Generative AI can automate the creation of marketing collateral such as ad copy, banners, and promotional videos. It can also analyse consumer sentiment and trends to optimize advertising campaigns and target audiences more effectively.
- Autonomous Vehicles: Autonomous vehicles utilise sophisticated artificial intelligence (AI) systems to function without human intervention. These AI algorithms process data from sensors to perceive their surroundings, identify objects, and navigate routes in real-time. By analysing sensor data, autonomous vehicles can anticipate hazards and adjust their trajectory to avoid collisions. Additionally, AI-driven predictive analytics enable these vehicles to predict maintenance needs, enhancing reliability and safety. As AI technology progresses, autonomous vehicles are expected to become increasingly commonplace, offering safer and more efficient transportation options.
- Manufacturing: In manufacturing, generative AI can optimize product design and manufacturing processes by generating and evaluating design alternatives. It can also predict equipment maintenance needs and identify opportunities for process improvements.
- Gaming and Entertainment: Generative AI contributes to dynamic and immersive gaming experiences by generating game levels, characters, narratives, and dialogue. Additionally, it can produce music, sound effects, and visual effects in gaming and entertainment media.
- Personalised Recommendations: Leveraging advanced machine learning algorithms, generative AI delivers highly tailored recommendations customized to individual preferences and behaviour patterns. Beyond product suggestions, it extends to personalized workout plans, meal recommendations, and learning resources, enhancing user engagement and satisfaction.
- Virtual Assistants and Chatbots: Generative AI powers virtual assistants and chatbots that engage in natural language conversations with users. These assistants can answer questions, provide assistance, make recommendations, and perform tasks on behalf of users.
- Content Creation: Generative AI streamlines content creation by autonomously generating text, images, videos, and other media forms. From crafting product descriptions to crafting engaging social media posts and blog articles, it ensures a consistent and compelling online presence effortlessly.
- Art and Design: In the realm of art and design, generative AI serves as a catalyst for creativity, aiding artists and designers in producing original artworks and designs with precision. This empowers them to explore new horizons of creativity and innovation, redefining artistic boundaries and inspiring groundbreaking creations.
Additionally, Generative AI showcases its prowess in various specific applications:
- Image Creation: Utilising methodologies such as Generative Adversarial Networks (GANs), Generative AI possesses the ability to craft lifelike images depicting objects, scenery, or even human faces that do not actually exist in the physical world.
- Text Generation: Advanced language models like GPT-3 demonstrate remarkable proficiency in generating text that closely resembles human language. These models can produce stories, poems, and articles when provided with specific prompts.
- Musical Composition: Generative AI showcases its talent by composing original pieces of music spanning various styles and genres, contributing to the creative landscape of music production.
- Video Synthesis: AI-driven systems have the capability to synthesise realistic videos, exemplified by technologies like deepfake, which pose ethical concerns due to their potential for misuse.
- Drug Development Assistance: Generative AI plays a pivotal role in drug discovery by designing new molecules with tailored properties, streamlining and expediting the process of developing new pharmaceuticals.
Generative AI vs Traditional AI
The primary difference between traditional AI and generative AI lies in their functionality and practical applications. Traditional AI systems are primarily focused on tasks related to data analysis and prediction, whereas generative AI goes a step further by generating new data similar to its training data.
In simple terms, traditional AI is adept at identifying patterns, while generative AI excels at creating new patterns. While traditional AI can analyze data and provide insights based on existing patterns, generative AI can use the same data to produce entirely novel content.
Key Contrasts
Now, let's explore the fundamental differences between traditional AI and generative AI:
Functionality:
- Generative AI: This type of AI comprehends context and can create various types of content that resemble human-like output, including text, code, music, audio, video, and more.
- Traditional AI: Conversely, traditional AI relies on patterns found in historical data to make predictions, usually in numerical form.
Applications:
- Generative AI: It finds diverse applications across different fields, capable of handling complex tasks and generating entirely new content like images, audio files, and videos.
- Traditional AI: On the other hand, traditional AI is usually tailored to specific tasks, such as detecting fraudulent activities, playing chess, or identifying anomalies in images.
Training Data:
- Generative AI: To learn and generate content, generative AI often uses extensive and varied datasets collected from the internet.
- Traditional AI: In contrast, traditional AI is trained using meticulously selected datasets designed for specific purposes.
Delivery Methods:
- Generative AI: It primarily interacts with humans through interfaces like chatbots on apps and web browsers.
- Traditional AI: Traditional AI is typically embedded in specialised applications tailored to particular use cases, such as generating business intelligence reports or managing call centre systems.
User Accessibility:
- Generative AI: Due to its broader applicability and user-friendly interfaces, generative AI is accessible to a wider range of users.
- Traditional AI: Conversely, traditional AI usually requires users to possess specialised knowledge and skills to effectively utilise it.
Conclusion
As we continue to journey through the dynamic realm of artificial intelligence, it becomes increasingly vital for organisations to acknowledge the profound impact of Generative AI on their operations and the evolving requirements of data centres. With its capability to generate diverse content and spur innovation, Generative AI is reshaping industries at a rapid pace. Therefore, it is imperative for businesses to reassess their data centre strategies, ensuring they accommodate the infrastructure demands of AI. Whether by optimising existing resources or seeking expert guidance to navigate the complexities of AI infrastructure, organisations must proactively embrace Generative AI to foster sustainable growth and maintain a competitive edge.
Connect with NEXTDC today to learn how we can help you navigate the evolving landscape of AI infrastructure and optimise your data centre strategy for the future.
Why Choose NEXTDC for Your Data Centre Needs?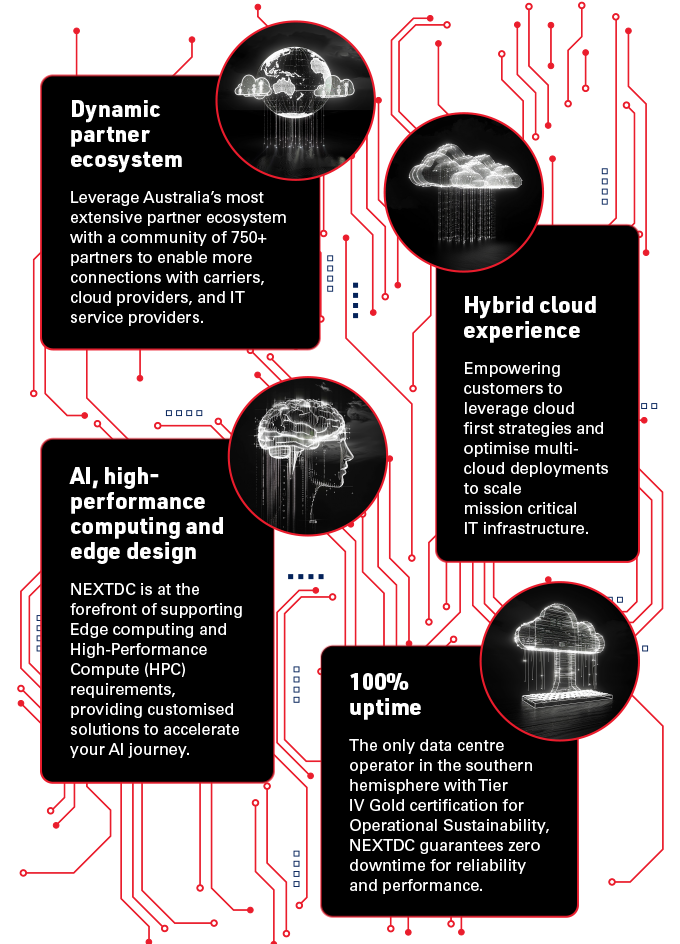